Three recently published studies focused on using artificial intelligence (AI) neural networks to generate audio output from brain signals have shown promising results, namely by producing identifiable sounds up to 80% of the time. Participants in the studies first had their brain signals measured while they were either reading aloud or listening to specific words. All the data was then given to a neural network to “learn” how to interpret brain signals after which the final sounds were reconstructed for listeners to identify. These results represent hopeful prospects for the field of brain-computer interfaces (BCIs), where thought-based communication is quickly moving from the realm of science fiction to reality.
The idea of connecting human brains to computers is far from new. In fact, several relevant milestones have been made in recent years including enabling paralyzed individuals to operate tablet computers with their brain waves. Elon Musk has also famously brought attention to the field with Neuralink, his BCI company that essentially hopes to merge human consciousness with the power of the Internet. As brain-computer interface technology expands and develops new ways to foster communication between brains and machines, studies like these, originally highlighted by Science Magazine, will continue demonstrating the steady march of progress.
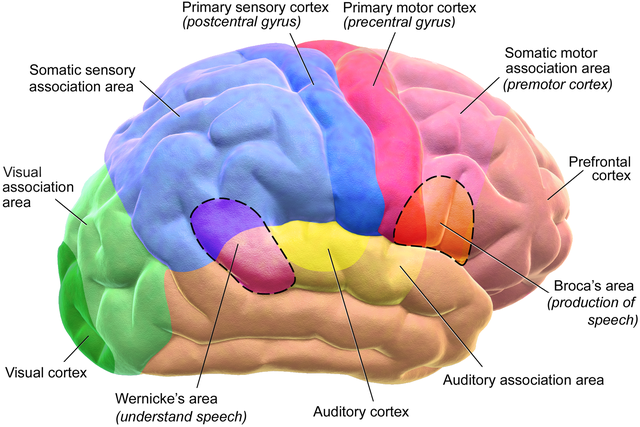
In the first study conducted by researchers from Columbia University and Hofstra Northwell School of Medicine, both in New York, five epileptic participants had the brain signals from their auditory cortexes recorded as they listened to stories and numbers being read to them. The signal data was provided to a neural network for analysis which then reconstructed audio files that were accurately identified by participating listeners 75% of the time.
In the second study conducted by a team from the University of Bremen (Germany), Maastricht University (Netherlands), Northwestern University (Illinois), and Virginia Commonwealth University (Virginia), brain signal data was gathered from six patients’ speech planning and motor areas while undergoing tumor surgeries. Each patient read specific words aloud to target the data collected. After the brain data and audio data were given to their neural network for training, the program was given brain signals not included in the training set to recreate audio, the result producing words that were recognizable 40% of the time.
Finally, in a third study by a team at the University of California, San Francisco, three participants with epilepsy read text aloud while brain activity was captured from the speech and motor areas of their brains. The audio generated from their neural network’s analysis of the signal readings was presented to a group of 166 people who were asked to identify the sentences from a multiple choice test – some sentences were identified with 80% accuracy.
While the research presented in these studies shows serious progress towards connecting human brains to computers, there are still a few significant hurdles. For one, the way neuron signal patterns in the brain translate into sounds varies from person to person, so neural networks must be trained on each individual person. The best results require the best data possible, i.e., the most precise neuron signals possible, meaning this is something that can only be obtained by placing electrodes in the brain itself. The opportunities to collect data at this invasive level for research are limited, relying on voluntary participation and approval of experiments.
All three of the studies highlighted demonstrated an ability to reconstruct speech based on neural data in some significant capacity; however, also in all cases, the study participants were able to create audible sounds to use with the computer training set. In the case of patients unable to speak, the level of difficultly in interpreting the brain’s speech signals from other signals will be the biggest challenge. Also, the differences between brain signals during actual speech vs. thinking about speech will complicate matters further.
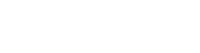