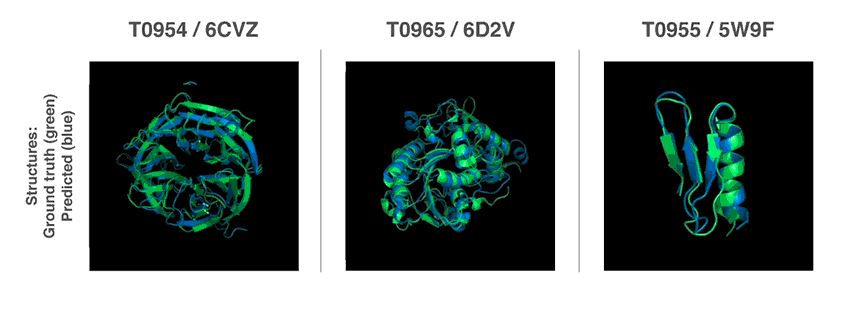
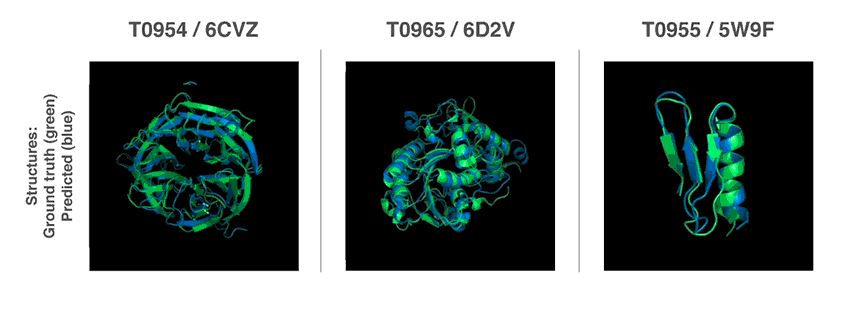
Energy
Google’s neural network takes a step closer to predicting disease using DNA
If humans had the ability to predict protein structure solely from DNA information, it would be a medical superpower against disease, and artificial intelligence is our best hope thus far to obtain it. Such a feat is now one step closer with the creation of “AlphaFold”, a neural network designed by Google’s AI company DeepMind, to do that very thing. After entering a biannual protein folding prediction contest called the Critical Assessment of Structure Prediction (CASP), AlphaFold was declared winner out of 98 AI competitors, specifically by most accurately predicting 25 of 43 protein shapes given using genetic sequences alone. The second place winner predicted only three.
In a nutshell (or smaller, really), proteins are key factors in every living thing’s physiological processes. Their structures are encoded in DNA, and they are responsible for contracting muscles, metabolizing food into energy, fighting disease, and transmitting signals, among a great many other things. The function of proteins depends on their unique 3D structure. The way they are shaped is directly related to what they do in the body. For example, antibodies have “hooks” that attach and tag viruses and bacteria, and ligament proteins are cord-shaped, enabling them to transmit tension.
The being said, the ability to predict protein shapes can enable scientists to learn more about how defects specifically affect the body, repair damaged ones with targeted therapies, and design new ones. Their specific structure is key – the 3D shape determines a protein’s function. To further illustrate this importance, misfolding proteins are linked to many health issues such as type 2 diabetes and Parkinson’s disease.
Some medical progress has been made to address protein folding issues such as drug therapies that bind to proteins and alter their function; however, the human body is able to generate around 2 million different types of proteins, and so far we can only identify about 100,000 of them. Out of those proteins, the variety of folded 3D structures possible is calculated to be a googol cubed – 10 to the power of 300. Clearly, this is not really a job for a human. As further described on DeepMind’s website, “[According to] Levinthal’s paradox, it would take longer than the age of the universe to enumerate all the possible configurations of a typical protein before reaching the right 3D structure.”
DeepMind is no stranger to achieving incredible things with its AI software. A program built by the company called “agent” learned to play 49 different retro computer games in 2015, making it the first computer program capable of independently learning a large variety of tasks. Two other programs named “AlphaZero” and “AlphaGo” were able to beat the world’s best human and computer players at chess and the ancient Chinese game “Go”, respectively. AlphaGo was later revised as “AlphaGo Zero” to play the same Go game without any prior human knowledge, i.e., it taught itself to play and subsequently win.
AlphaFold was trained with thousands of known proteins until it could accurately predict those proteins’ 3D shape. This was a significant improvement over other existing technology, not only in levels of accuracy, but in cost-effectiveness. Other protein identification techniques such as cryo-electron microscopy and nuclear magnetic resonance depend on a lot of trial and error, which involves years of work and several thousands of dollars per protein structure to achieve. Considering the complexity involved in this field, the AlphaFold’s achievement in the CASP contest is, to say the least, representative of the expanding possibilities for scientific research and discovery using artificial intelligence.
Energy
Tesla Lathrop Megafactory celebrates massive Megapack battery milestone
The Tesla Megapack is the backbone of Tesla Energy’s battery deployments.
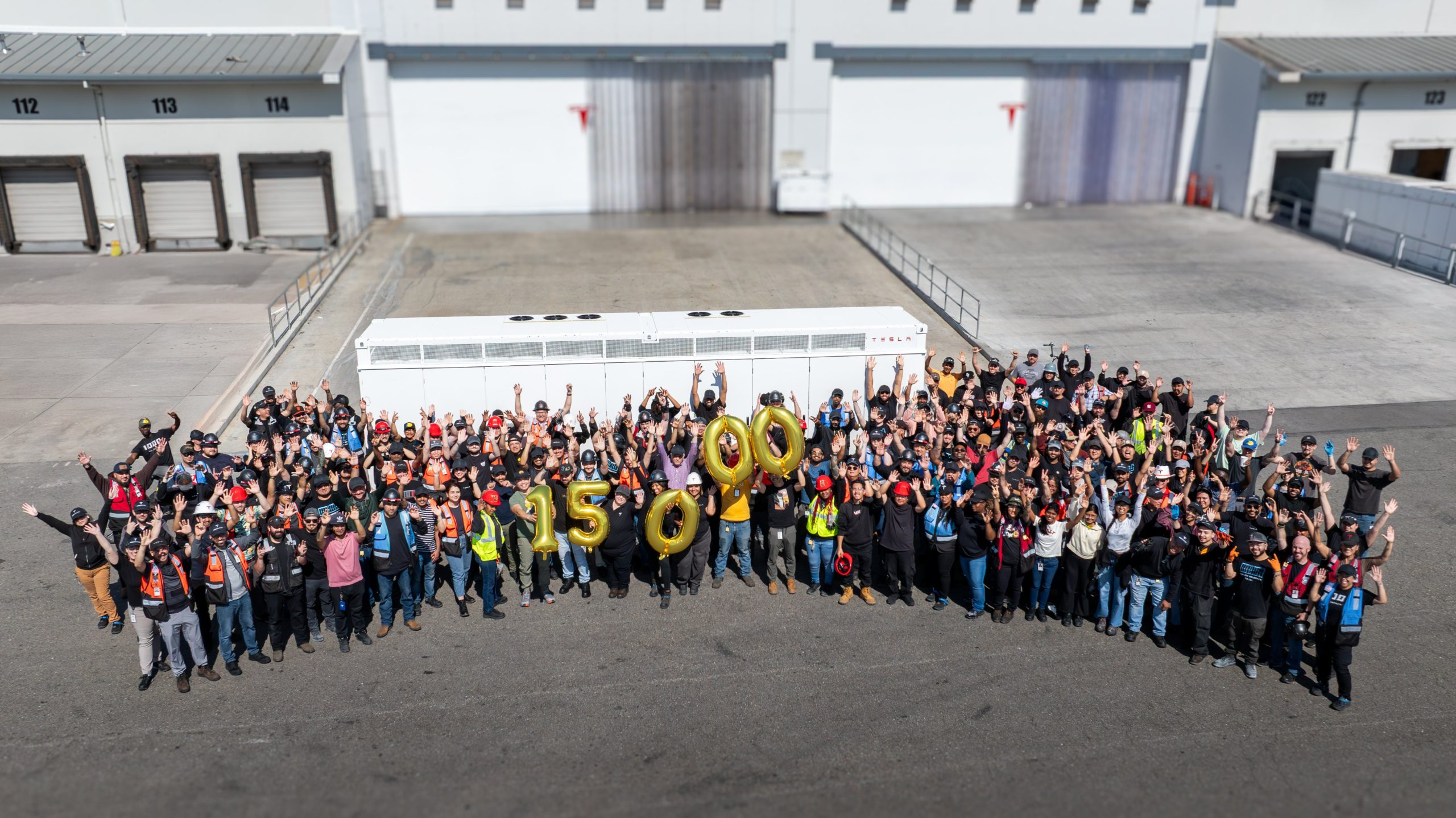
The Tesla Lathrop Megafactory recently achieved a new milestone. As per the official Tesla Megapack account on X, the Lathrop Megafactory has produced its 15,000th Megapack 2 XL battery.
15,000 Megapack Batteries
Tesla celebrated the milestone with a photo of the Lathrop Megafactory team posing with a freshly produced Megapack battery. To commemorate the event, the team held balloons that spelled out “15,000” as they posed for the photo.
The Tesla Megapack is the backbone of Tesla Energy’s battery deployments. Designed for grid-scale applications, each Megapack offers 3.9 MWh of energy and 1.9 MW of power. The battery is extremely scalable, making it perfect for massive energy storage projects.
More Megafactories
The Lathrop Megafactory is Tesla’s first dedicated facility for its flagship battery storage system. It currently stands as the largest utility-scale battery factory in North America. The facility is capable of producing 10,000 Megapack batteries every year, equal to 40 GWh of clean energy storage.
Thanks to the success of the Megapack, Tesla has expanded its energy business by building and launching the Shanghai Megafactory, which is also expected to produce 40 GWh of energy storage per year. The ramp of the Shanghai Megafactory is quite impressive, with Tesla noting in its Q1 2025 Update Letter that the Shanghai Megafactory managed to produce over 100 Megapack batteries in the first quarter alone.
Tesla Energy’s Potential
During the first quarter earnings call, CEO Elon Musk stated that the Megapack is extremely valuable to the energy industry.
“The Megapack enables utility companies to output far more total energy than would otherwise be the case… This is a massive unlock on total energy output of any given grid over the course of a year. And utility companies are beginning to realize this and are buying in our Megapacks at scale,” Musk said.
Energy
Tesla Megapacks powers the xAI Colossus supercomputer
Tesla Megapacks step in to stabilize xAI’s Colossus supercomputer, replacing natural gas turbines. Musk’s ventures keep intertwining.
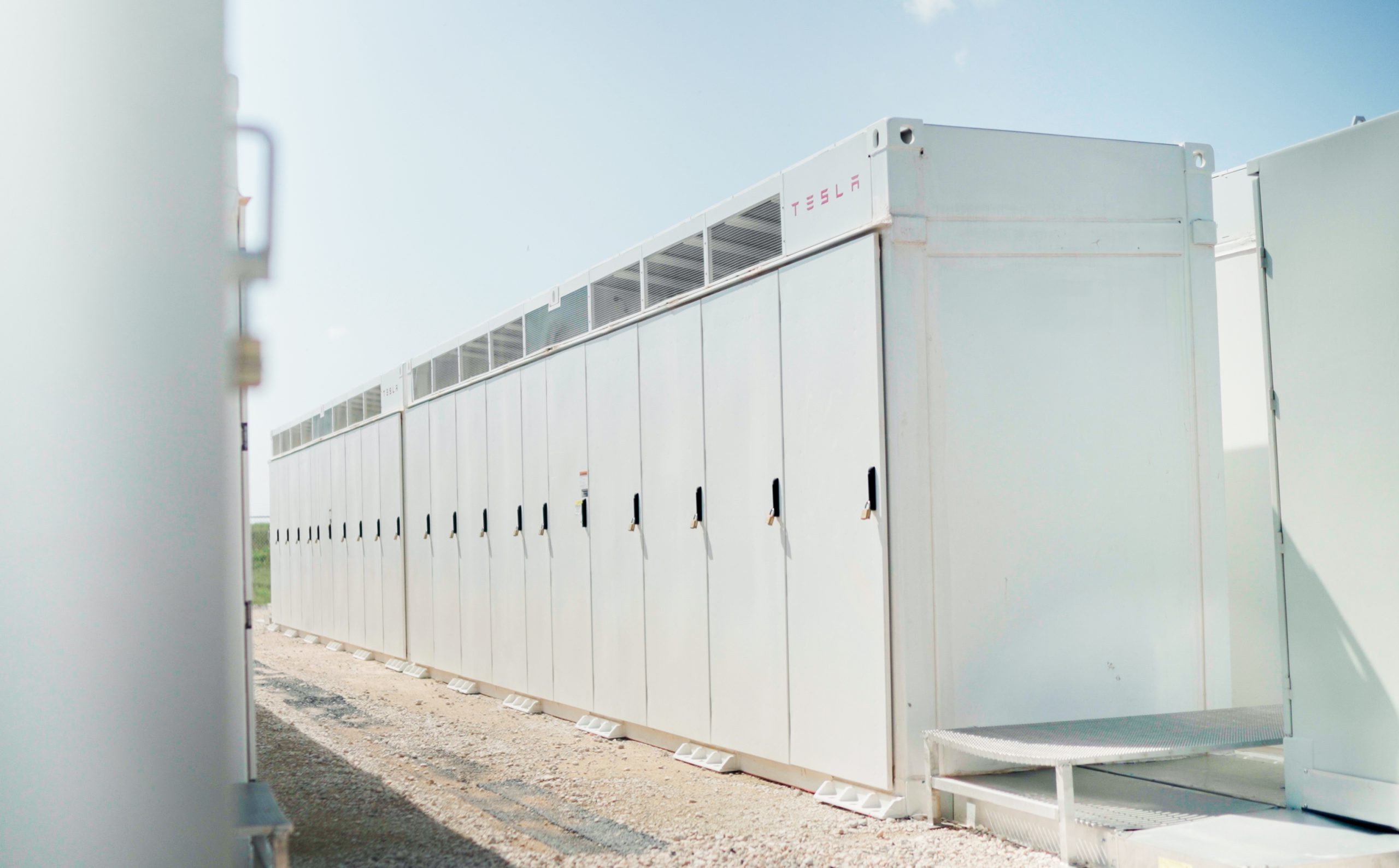
Tesla Megapack batteries will power the xAI Colossus supercomputer in Memphis to ensure power stability. The collaboration between Tesla and xAI highlights the synergy among Elon Musk’s ventures.
The artificial intelligence startup has integrated Tesla Megapacks to manage outages and demand surges, bolstering the facility’s reliability. The Greater Memphis Chamber announced that Colossus, recently connected to a new 150-megawatt electric substation, is completing its first construction phase. This transition addresses criticism from environmental justice groups over the initial use of natural gas turbines.
“The temporary natural gas turbines that were being used to power the Phase I GPUs prior to grid connection are now being demobilized and will be removed from the site over the next two months.
“About half of the operating turbines will remain operating to power Phase II GPUs of xAI until a second substation (#22) already in construction is completed and connected to the electric grid, which is planned for the Fall of 2025, at which time the remaining turbines will be relegated to a backup power role,” the Chamber stated.
xAI’s rapid development of Colossus reflects its ambition to advance AI capabilities, but the project has faced scrutiny for environmental impacts. The shift to Megapacks and grid power aims to mitigate these concerns while ensuring operational continuity.
The Megapack deployment underscores the collaboration among Musk’s companies, including Tesla, SpaceX, Neuralink, and The Boring Company. Tesla appears to be the common link between all of Musk’s companies. For example, The Boring Company built a tunnel in Giga, Texas. In addition, Musk has hinted at a potential collaboration between the Tesla Optimus Bot and Neuralink. And from January 2024 to February 2025, xAI invested $230 million in Megapacks, per a Tesla filing.
Tesla Energy reported a 156% year-over-year increase in Q1 2025, deploying 10.4 GWh of storage products, including Megapacks and Powerwalls. Tesla’s plans for a new Megapack factory in Waller County, Texas, which is expected to create 1,500 jobs in the area, further signal its commitment to scaling energy solutions.
As xAI leverages Tesla’s Megapacks to power Colossus, the integration showcases Musk’s interconnected business ecosystem. The supercomputer’s enhanced stability positions xAI to drive AI innovation, while Tesla’s energy solutions gain prominence, setting the stage for broader technological and economic impacts.
Energy
Tesla Energy celebrates one decade of sustainability
Tesla Energy has gone far since its early days, and it is now becoming a progressively bigger part of the company.
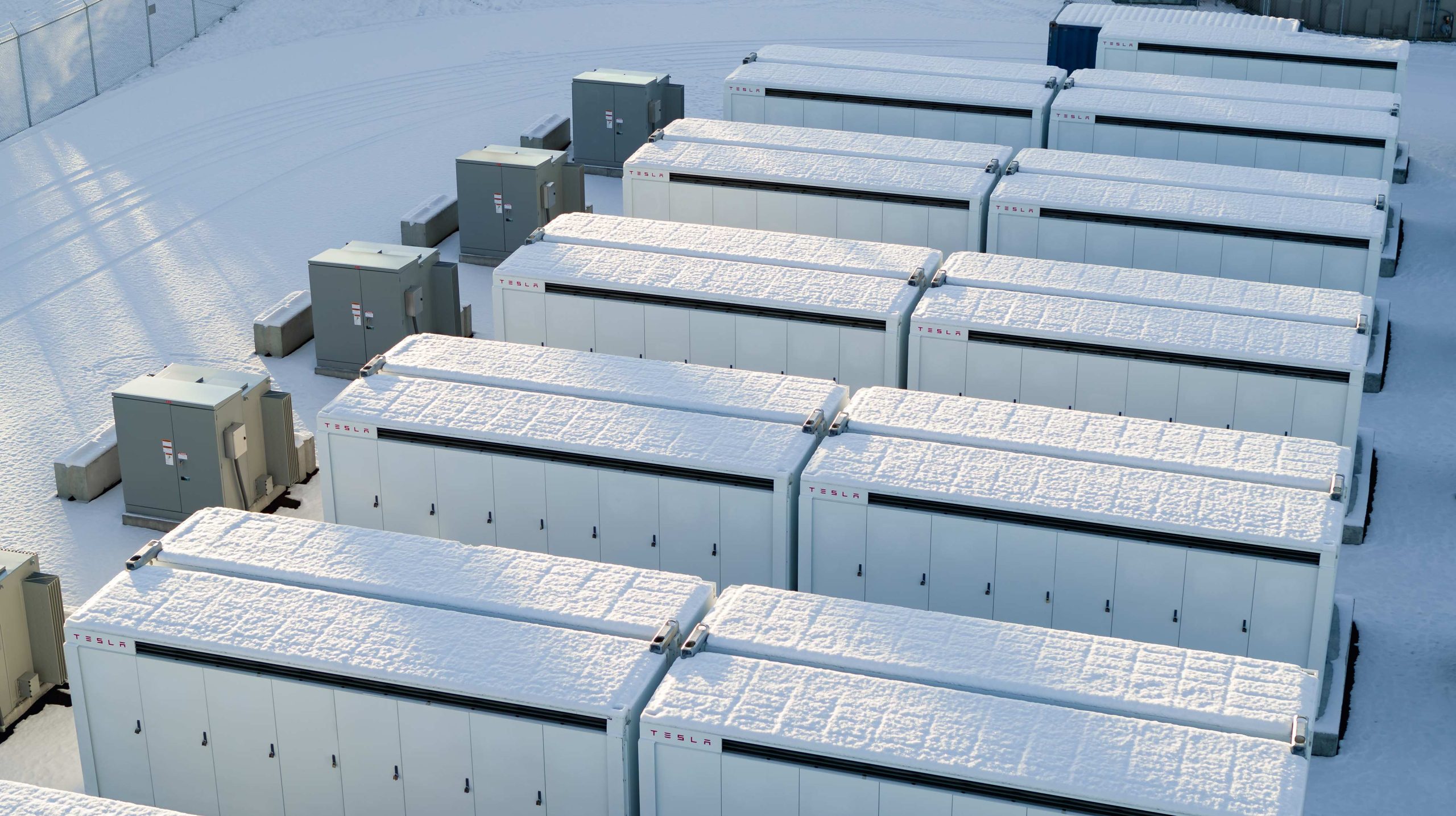
Tesla Energy recently celebrated its 10th anniversary with a dedicated video showcasing several of its milestones over the past decade.
Tesla Energy has gone far since its early days, and it is now becoming a progressively bigger part of the company.
Tesla Energy Early Days
When Elon Musk launched Tesla Energy in 2015, he noted that the business is a fundamental transformation of how the world works. To start, Tesla Energy offered the Powerwall, a 7 kWh/10 kWh home battery system, and the Powerpack, a grid-capable 100 kWh battery block that is designed for scalability. A few days after the products’ launch, Musk noted that Tesla had received 38,000 reservations for the Powerwall and 2,500 reservations for the Powerpack.
Tesla Energy’s beginnings would herald its quiet growth, with the company later announcing products like the Solar Roof tile, which is yet to be ramped, and the successor to the Powerwall, the 13.5 kWh Powerwall 2. In recent years, Tesla Energy also launched its Powerwall 3 home battery and the massive Megapack, a 3.9 MWh monster of a battery unit that has become the backbone for energy storage systems across the globe.
Key Milestones
As noted by Tesla Energy in its recent video, it has now established facilities that allow the company to manufacture 20,000 units of the Megapack every year, which should help grow the 23 GWh worth of Megapacks that have already been deployed globally.
The Powerwall remains a desirable home battery as well, with more than 850,000 units installed worldwide. These translate to 12 GWh of residential entry storage delivered to date. Just like the Megapack, Tesla is also ramping its production of the Powerwall, allowing the division to grow even more.
Tesla Energy’s Role
While Tesla Energy does not catch as much headlines as the company’s electric vehicle businesses, its contributions to the company’s bottom line have been growing. In the first quarter of 2025 alone, Tesla Energy deployed 10.4 GWh of energy storage products. Powerwall deployments also crossed 1 GWh in one quarter for the first time. As per Tesla in its Q1 2025 Update Letter, the gross margin for the Energy division has improved sequentially as well.
-
Elon Musk1 day ago
Tesla investors will be shocked by Jim Cramer’s latest assessment
-
News6 days ago
Tesla Robotaxi’s biggest challenge seems to be this one thing
-
News2 weeks ago
Tesla’s Grok integration will be more realistic with this cool feature
-
Elon Musk2 weeks ago
Elon Musk slams Bloomberg’s shocking xAI cash burn claims
-
News2 weeks ago
Tesla China roars back with highest vehicle registrations this Q2 so far
-
News2 weeks ago
Texas lawmakers urge Tesla to delay Austin robotaxi launch to September
-
News2 weeks ago
Tesla dominates Cars.com’s Made in America Index with clean sweep
-
Elon Musk1 week ago
First Look at Tesla’s Robotaxi App: features, design, and more